April 1, 2024
Students were recognized for their research that aims to improve robotics, batteries, disease diagnoses and more.
This winter, the ME department hosted its first annual student poster competition. The event, hosted by the Mechanical Engineering Graduate Student Association (MEGA), showcased more than 40 graduate students’ abstracts and was judged by faculty members. Congratulations to the following students who each received a $500 prize for their poster.
Emilee Armstrong (Materials, Mechanics, and Fluids award)
Poster title: Manufacturing high-Performance three-dimensional Lithium-ion battery electrode architectures using acoustophoresis
Adviser: Corie L. Cobb, Washington Research Foundation Innovation Professor in Clean Energy and ME Professor
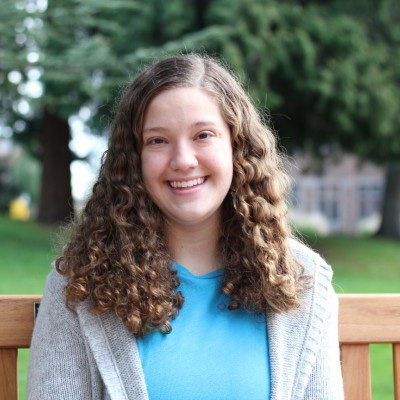
Emilee Armstrong
What is your project about?
My project lies at the intersection of physics and engineering, focusing on developing new manufacturing processes for Lithium-ion batteries that allow us to create higher performance batteries for electric vehicle (EV) applications. I am specifically leveraging acoustic forces to architect 3D structure in Lithium-ion battery electrodes to enable better battery performance at fast charge and discharge rates that are critical for EVs.
What excites you about this research?
Developing a new manufacturing process for Lithium-ion battery electrodes has been a great experience. I greatly appreciate that funding from the U.S. Department of Energy’s Advanced Materials and Manufacturing Technologies Office (AMMTO) has supported our team and enabled us to develop a new scalable manufacturing process for 3D structured electrodes. Moreover, we are striving to develop manufacturing processes that are not only effective at producing high-energy batteries with fast charging capabilities, but that are also readily adaptable to new battery materials and a wide range of battery applications. In the dynamic field of Lithium-ion batteries, this manufacturing adaptability is crucial to harnessing the full potential of new materials.
Gan Gao (Health award)
Poster title: Deep-learning triage of 3D pathology datasets for more accurate detection of esophageal neoplasia while reducing pathologist workloads
Adviser: ME Professor Jonathan Liu
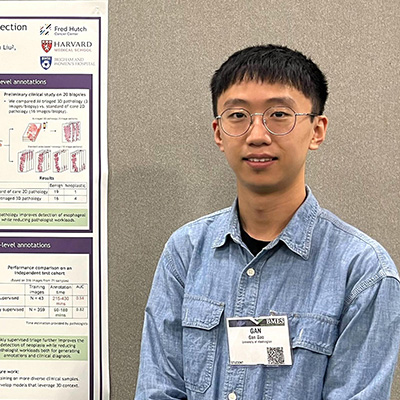
Gan Gao
Could you provide a brief description of your research?
Early detection of esophageal dysplasia and cancer in patients with Barrett’s esophagus, a complication of gastrointestinal reflux disease, is key for patient survival. Unfortunately, the assessments of biopsies obtained during regular surveillance endoscopy of Barrett’s esophagus patients are not ideal for detecting dysplasia and cancer. Specifically, only a few thin tissue sections from each biopsy are examined with standard-of-care 2D pathology methods, representing less than 1% of the biopsies.
Comprehensive evaluation of whole biopsies with non-destructive 3D pathology may improve the sensitivity of detecting dysplasia and cancer. However, the large datasets generated by 3D pathology can be tedious for pathologists to manually review. Therefore, we developed a deep-learning-based triage method to automatically identify 2D image sections that are most likely to contain dysplasia and cancer within 3D pathology datasets for pathologist assessment, in order to improve disease detection while reducing pathologist workloads.
What excites you about the project?
I'm particularly excited about the potential impact this project could have on clinical practice. For example, we conducted a preliminary clinical validation with UW Medicine. In this study, we compared the diagnosis of 20 biopsies using AI-triaged images from 3D pathology data against the standard-of-care 2D histology.
The preliminary results are incredibly promising. Our deep-learning triaged 3D pathology workflow identified dysplasia in 3 biopsies that would have otherwise been missed by 2D histology. The results suggest our work can potentially enhance the detection of esophageal cancer and dysplasia, enabling earlier intervention and improving patient outcomes, while also reducing workloads for pathologists — a win-win situation.
Ian Good (Mechatronics and Controls award)
Poster title: Enhancing the performance of Pneu-net actuators using a torsion resistant strain limiting layer
Advisers: ME Assistant Professor Aniruddh Vashisth and Northeastern University Assistant Professor Jeffrey Lipton
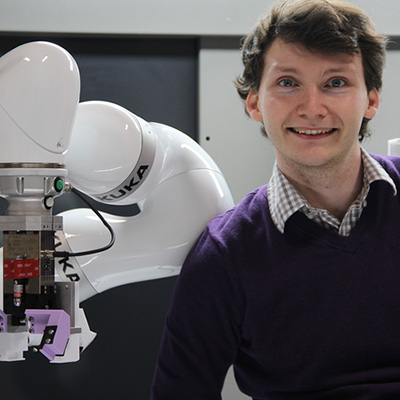
Ian Good
What is your project about?
We took a soft gripper that struggled to pick up 50 grams, and made it lift five kilograms. This poster looks at ways we can make soft robots, or robots that are squishy like humans, better able to lift objects. These soft grippers are called Pneumatic Network (Pneunet) Grippers and are the most common soft robotic gripper. They have two components: an air bladder, and a strain limiting layer. We saw that the Pneunet grippers struggled to lift objects due to unfavorable twisting. We created mathematical equations that described soft gripper twisting and used them to come up with a better strain limiting layer design. Our design reduced the unfavorable twisting by 97.7%, and increased the maximum weight the gripper could lift to five kilograms, the maximum weight our robot arm could support.
What excites you about this research?
I am excited to make robots that make the world a better place. As a first-generation college student with a parent in sheet metal construction, I grew up with an awe of the structures my parents made. As I aged, the realities of my family’s physically demanding jobs set in. Through robots and automation, I believe we can reduce the burden on physically demanding jobs while multiplying societal good. In my work, I use material and structure to solve problems in robots. I work on projects around gripping, hopping and manipulating objects. This work excites me because we were able to take a gripper from lifting strawberries to lifting 10-pound dumbbells. One day soon, I hope the robots I build can come out of the lab and start making the world a better place.
Niharika Karnik (People's Choice award)
Poster title: Constrained optimization of sensor placement for nuclear digital twins
Adviser: ME Assistant Professor Krithika Manohar
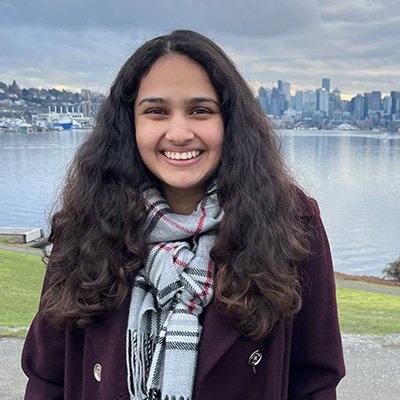
Niharika Karnik
What is your research about?
The deployment of extensive sensor arrays in nuclear reactors is infeasible due to challenging operating conditions and inherent spatial limitations. Optimal placement of sensors with respect to restrictive spatial constraints is essential for the reconstruction of nuclear reactor flow fields and online calibration of nuclear digital twins. My research focuses on developing a data-driven optimization technique for sensor placement, which aims to minimize reconstruction error of flow fields under sparse, noisy sensor measurements. The proposed greedy algorithm optimizes sensor locations over high-dimensional grids, adhering to spatial and dynamic constraints based on operating tolerances of sensors. We apply the algorithm to the Out-of-Pile Testing and Instrumentation Transient Water Irradiation System (OPTI-TWIST) prototype capsule, which is electrically heated to emulate the neutronic effect of the nuclear fuel. The resulting sensor-based temperature reconstruction within OPTI-TWIST demonstrates minimized error, provides probabilistic bounds for noise-induced uncertainty, and establishes a foundation for communication between the digital twin and the experimental facility.
What excites you about this research?
I am very excited to apply data-driven approaches to traditional engineering and safety critical systems such as nuclear fuel rodlets. I love analyzing patterns in data from models and simulations of real-world systems to establish mathematical models that capture the dominant behaviors of these systems. I enjoy coding challenges that come along with the implementation of each new data-driven technique and dataset. I am happy to contribute to the formation of nuclear digital twins which would help design and license nuclear power plants through detailed safety and reliability analyses.